Conclusion
-
Feature Analysis
In the data preprocess, some interesting relationships among different features are revealed:
-
The top three features related to Purchase is: Product_Category_1, Product_ID and User_ID.
​
​
​
​
​
​
​
​
​
​
2. Among customer demographics, Occupation is the most related to Purchase, other attributes such as gender/marital_status/stay_years has no significant correlation.
-
ML model Evaluation
-
For task 1, all attributes are considered to predict Purchase. When implementing Collaborative Filtering wrote by ourselves with cosine similarity and k_neighbor = 3, the average RMSE for predicting Purchase in 10-Folds-Cross validation is 3361.723. However, using Random Forest model with n_estimators = 100, the RMSE for predicting Purchase in 10-Folds-Cross validation is 2932. Considering the code by ourselves is not as efficiency as sklearn package, this results is acceptable.
-
For task2, only customer demographics can be used. From features analysis, we know that customer-specific and product-specific information are most related to Purchase, therefore, it's hard to expect good prediction. The highest accuracy with SVM, DT and KNN is 51.3% when predicting Purchase which have been discretized to 1~4.
​
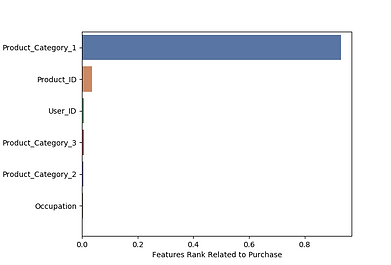
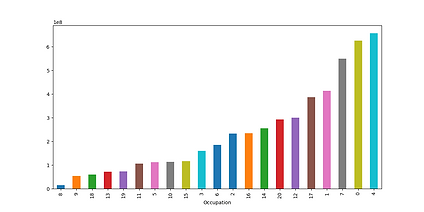
